Our project trains the InceptionV3 machine learning model for the morphological classification of galaxies using the dataset from the Galaxy Challenge by Galaxy Zoo. The motivation for applying machine learning algorithms to this problem is to relieve astrophysicists from the repetitive and time-consuming task of manually classifying galaxies. By leveraging the power of InceptionV3, a sophisticated convolutional neural network, we can automate this process, enabling faster and more accurate analysis of galaxy images. This approach not only enhances efficiency but also allows scientists to focus on more complex and interpretative aspects of their research.

Using a dataset of crowdsourced answers to 11 different metrics, we trained our model to classify galaxies into 37 detailed morphological categories. The InceptionV3 model demonstrated significant improvements in accuracy over baseline models, underscoring the potential of machine learning in advancing astronomical research. This project not only contributes to the field of astrophysics but also provides valuable insights into the application of neural networks for complex data classification tasks. As we continue to refine our model and expand our dataset, we look forward to further unlocking the mysteries of the universe through the lens of machine learning.
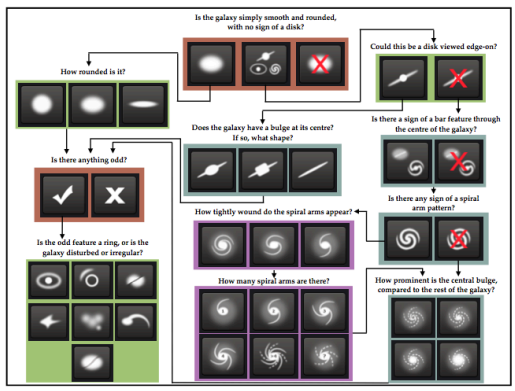
If you are interested in learning more about this project, you can watch our presentation
below!